Tech World
How are Silicon Valley professionals reducing AI hallucinations?
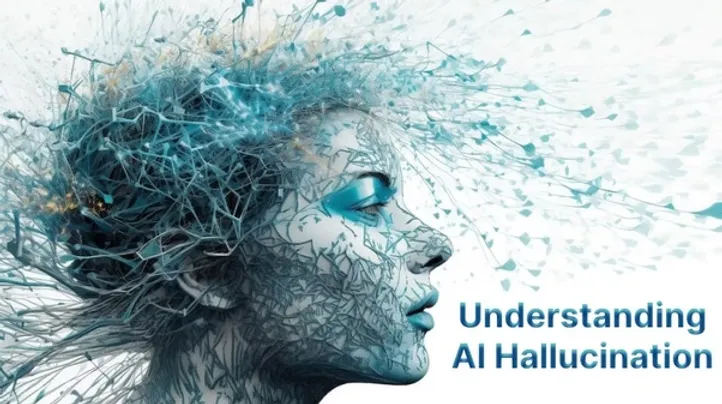
Generative AI, also known as Gen AI, represents a form of artificial intelligence capable of creating original content such as text, art, and music by leveraging existing online content. However, despite its capabilities, Generative AI is susceptible to inaccuracies or “hallucinations.” These occur when the AI generates responses that are factually incorrect or unverifiable, akin to a person seeing things that aren’t real.
To mitigate these challenges, Silicon Valley innovators have devised strategies, with Retrieval Augmented Generation (RAG) emerging as a prominent solution, as reported by Wired.
Unlike traditional approaches where AI models rely solely on their training data, RAG enhances queries by sourcing information from a specialized database before generating responses using a Large Language Model (LLM). This method aims to anchor AI-generated content in verified sources, reducing the incidence of hallucinations.
Pablo Arredondo, vice president of CoCounsel at Thomson Reuters, explained to Wired that RAG improves accuracy by integrating “real documents” relevant to the query topic, ensuring the AI’s responses are grounded in reliable data.
Despite its effectiveness, RAG isn’t foolproof, and instances of AI hallucinations may still occur. Factors influencing its success include the quality of the database used, the precision of the search process, and the relevance of the retrieved information to the query.
Ultimately, the goal is to ensure that outputs from Generative AI models are both grounded in factual data and accurate, reinforcing reliability in AI-generated content.
